Elena Black, PhD, FSA, EA, CFA, MAAA
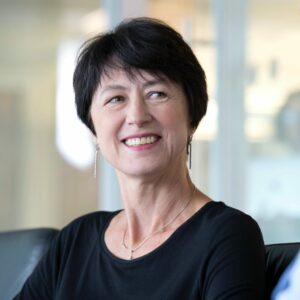
Elena (Lena) Black is a Senior Research Actuary at the Terry Group, an actuarial and analytics consulting firm.
Lena earned her PhD in mathematics from University of Maryland, College Park. Lena’s academic career began with postdoc positions at the University of Pennsylvania and at the Mathematical Sciences Research Institute at Berkeley and continued with a tenure track position at the University of Oklahoma. During her academic career, Lena taught a variety of undergraduate and graduate mathematics courses, conducted research, and published academic research papers in algebraic geometry.
Following her academic career, Lena pursued her actuarial credentials and earned her CFA® charterholder designation. She is a Fellow of Society of Actuaries, an Enrolled Actuary, and a Member of the American Academy of Actuaries.
As a leading pension practitioner and researcher, Lena has developed a variety of financial management tools and approaches, including the construction of yield curves, liability-driven investment strategies, financial modeling and asset liability management tools. Her actuarial research interests into such areas as demography, longevity and relevant credibility theory has landed her in key leadership roles in the actuarial profession supporting industry-wide mortality models and public policy development in pensions and related fields. She has been an active volunteer with the Society of Actuaries and the American Academy of Actuaries.
The Power of Back-testing
As a practical matter, actuaries use models in many everyday tasks, including actuarial valuations, asset liability management studies, healthcare risk scoring, pension plan re-design, and many others. One of the ways of evaluating a model is to examine how it would have worked had it been implemented in the past. Simple in concept, but too rarely used in practice, back-testing is a powerful tool for validating a model’s strengths, but also for revealing its potential vulnerabilities.
In this presentation, Ms. Black demonstrates the insights that have been gleaned from back-testing in several real-world actuarial modeling exercises including yield curve construction, mortality improvement scales’ models, investment strategies such as liability driven investment or dynamic asset allocations, models for forecasting capital market assumptions, and healthcare risk scoring models.
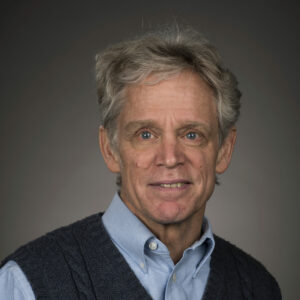
Edward (Jed) Frees, University of Wisconsin – Madison, Australian National University
Edward (Jed) Frees is an emeritus professor affiliated with the University of Wisconsin-Madison where he served as the Hickman Larson Chair of Actuarial Science. Since 2019, he has enjoyed a fractional research appointment with the Australian National University. He received his Ph.D. in mathematical statistics from the University of North Carolina at Chapel Hill and is a Fellow of both the Society of Actuaries (SOA) and the American Statistical Association (the only Fellow of both organizations).
Professor Frees has provided extensive service to the profession, including serving as the founding chairperson of the SOA Education and Research Section, a member of the SOA Board of Directors, a Trustee of the Actuarial Foundation, the Editor of the North American Actuarial Journal, and as an actuarial representative to the Social Security Advisory Board’s Technical Panel on Methods and Assumptions. He has written three books, edited a two-volume series on Predictive Modeling Applications in Actuarial Science, and is editing an online, open source book Loss Data Analytics.
Regarding his research, Professor Frees has published extensively and won several awards for his work. He has won the Society of Actuaries’ Annual Prize for best paper published by the Society, the SOA’s Ed Lew Award for research in modeling, the Casualty Actuarial Society’s Hachmeister award, and the Halmstad Prize for best paper published in the actuarial literature (four times).
Diversifying Insurable Risk Portfolios
Commercial organizations face many risks such as damage to their buildings due to fire, liability resulting from management misbehavior, and threats external to the organization such as cyber attacks. Firms have financial responsibility for these risks, they ‘own’ them, and so it is natural to refer to the collection of these many risks as a portfolio. Analogous to the familiar asset portfolio allocation methods, this talk demonstrates how risk managers can use constrained optimization algorithms to provide guidance on the amount of risk to retain or transfer. Using a case study, we are largely able to corroborate the work of expert risk brokers who make risk retention decisions using their extensive experience and knowledge of the worldwide insurance marketplace. Moreover, this insurance framework supplements their work by emphasizing the risk versus return/cost trade-off and offering data visualization educational tools that help improve managers’ financial literacy.
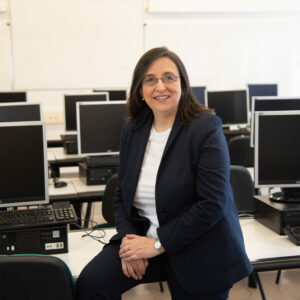
Montserrat Guillen, University of Barcelona
Montserrat Guillen is Chair Professor of Quantitative Methods at the University of Barcelona (UB), where she is the director of the RISKcenter. She is PhD in Economics, MSc in Mathematical Statistics and MA in Data Analysis. She is a top-cited author in Actuarial Science and Insurance. Chief-editor of the North American Actuarial Journal since January 2023. Optimistic, she likes understanding data and mitigating risks. She loves having friends visiting Barcelona from around the world.
Pricing Motor Insurance with Telematics Data
Many insurance companies collect telematics data about drivers’ exposure to traffic (distance driven and type of road), their driving behavior (excess speed, aggressiveness, operating hours) and contextual information (weather conditions, traffic intensity). Actuaries can use this information to improve motor insurance ratemaking. Several recent proposals will be presented, mostly using traditional GLM models and addressing weekly ratemaking. Personalized driving risk indicators can also promote driving safety. Illustrations with several real data sets provided by insurance companies will answer questions: (1) How are pay-per-mile insurance schemes be designed? (2) How can near-miss telematics be used to identify risky drivers? (3) Can external factors such as weather conditions be integrated?